Where Mathematics Meets Social Justice
By Tom PorterAs a former resident of Burlington, Vermont, Associate Professor of Mathematics Thomas Pietraho clearly recalls the controversy surrounding the mayoral election of 2009. “It was an example,” he said, “of how ranked choice voting can cause the most popular candidate to lose an election.”
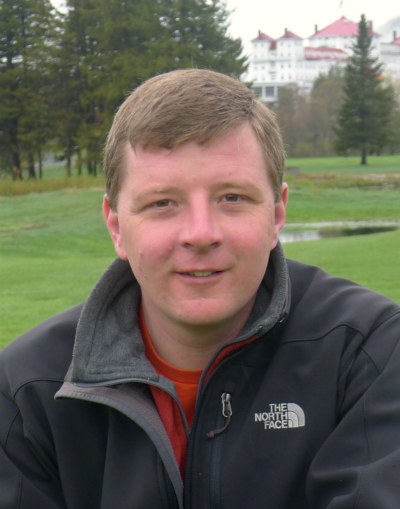
Not that any particular voting system is better than another, though, he added. They all have their imperfections. “Whatever system you use, there is going to be a set of circumstances where something undesirable can happen,” said Pietraho, citing a mathematical concept known as “Arrow's impossibility theorem.”
The challenges of finding the fairest voting method—in particular, how mathematicians might tackle those challenges—were discussed in depth recently at a seminar delivered by Visiting Assistant Professor of Mathematics Michael Ben Zvi '13. The event, called “What Is the Best Way to Pick a Winner,” was part of an ongoing series of seminars launched by the math department in September called “Math for the Common Good.”
The series, which includes four events this semester, features Bowdoin faculty and visiting speakers discussing a variety of subjects from politics to public health to the ethics of algorithms. The aim, said Pietraho, is to think about how mathematicians can tackle some of the social issues of the present day. “I think what’s happening in mathematics is that the field is widening, so it’s not just about the more traditional ‘hard science’-oriented issues like physics and engineering, but also includes questions that address the common good, such as ‘How do we design the perfect election?’ or ‘What’s the fairest way to distribute a vaccine?’ or ‘How can you design an algorithm that’s free from bias?’”
Prompted by the growth of the Black Lives Matter movement, said Pietraho, math faculty members started having conversations during the summer over how best to highlight ways in which math can inform questions of social justice. “We want to make sure our major is meaningful to as broad an audience as possible and that our students are aware of some of the problems they could work on with the skills they learn at Bowdoin.”
The series kicked off with a talk called “Modeling Infectious Disease Transmission for Public Health” by Sophie Berube '15, a researcher at John Hopkins University. “She studies infectious diseases and for the last few months has been looking almost exclusively at COVID-19.” Being a mathematician, Pietraho said, has enabled Berube to study how the disease has spread and what might be the best method for deploying a limited amount of vaccine in such a way as to have the biggest effect.
The final talk is on December 1, when Pietraho himself will address “A Mathematical Perspective on Algorithmic Bias.” “Algorithms are important, whether we like them or not,” he said. “At some point, there's going to be some sort of computer that will decide questions like ‘Should the following person receive parole?’ ‘Should this self-driving car slow down or accelerate at this point?’ The question we should be asking ourselves is ‘Are different populations affected equally by these machine-made suggestions?’”
There are a number of examples of how the use of algorithms has discriminated against certain people, he explained. “There was an example at Amazon a few years ago, when it was revealed that job offers were being made on the basis of a biased algorithm that showed a strong preference for male applicants. Applications from women,” said Pietraho, “were being filtered out before the first human laid eyes on them.”
"In mathematics... the field is widening, so it’s not just about the more traditional ‘hard science’-oriented issues like physics and engineering, but also includes questions that address the common good, such as ‘How do we design the perfect election?’ or ‘What’s the fairest way to distribute a vaccine?’”
Another example of algorithmic bias, said Pietraho, was found in the technology used in self-driving cars. “Cars have to decide between pedestrians and immovable objects, and it turns out that the color of your skin affects whether the algorithm thinks you are a pedestrian or not. Darker images are perceived as more likely to be immovable objects.”
The next talk this semester has a similar theme. Computer scientist Cameron Carpenter presents “An Introduction to Facial Recognition Algorithms” on November 17. These types of algorithms are used in law enforcement and criminal investigation, said Pietraho, and how to use them fairly is an enormously complicated question. “Any algorithms we deploy as a society, and especially ones that inform decisions to arrest and prosecute, must not only be accurate, but also treat all populations equitably.”
Concern over the accuracy and fairness of facial recognition algorithms has prompted a number of police departments to stop using them. Other algorithms too are being pulled from the shelves, and Pietraho is worried that society is becoming very wary of anything called an algorithm. “This technology, as well as having huge potential for bias, also has huge potential to help us make better decisions as a society. This is why this it’s such an important area for math scholars to study,” he said.