Bowdoin Effort Earns Top Award at International Computer Science Conference
By Tom Porter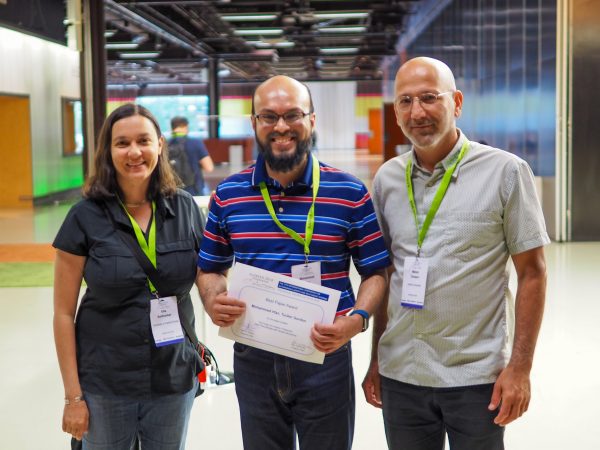
A research paper coauthored by a Bowdoin professor and one of his former students has earned the top spot at a recent computer science conference in Sweden. The paper employs computational game theory to model and predict congressional voting patterns. It was written by Assistant Professor of Digital and Computational Studies and Computer Science Mohammad Irfan and Tucker Gordon ’17, who now works as a data scientist at the technology consulting firm Booz Allen Hamilton.
The study, which grew out of Gordon’s honors project, won the best paper award at the 17th International Conference on Autonomous Agents and Multiagent Systems (AAMAS) in Stockholm, one of the premier, peer-reviewed conferences in this particular field of artificial intelligence (AI), said Irfan. “I learned that close to 600 papers were reviewed this year and the top 25 percent were accepted by the conference as full papers. Each paper gets three double-blind reviews,” he said, “so just getting it accepted in such a competitive environment was a big deal. To get the ultimate award was simply incredible.”
Irfan, who traveled to Stockholm to present the paper, said the achievement is a testament to the type of students he encounters at Bowdoin. “Even as undergraduates,” he said, “they are mature and competent enough to perform extremely high-quality research.” The paper that Irfan and Gordon authored—”The Power of Context in Networks: Ideal Point Models with Social Interactions”—models strategic behaviors in a social network while considering the underlying behavioral context in the congressional setting. This enables a more accurate voting prediction than previous models by utilizing some contextual information.
To quote from the paper itself: “The challenges in modeling and predicting how legislators vote come in identifying patterns in data, constructing models of voting behavior, and using these models to make predictions on future votes.” The study goes on to say: “a model of legislative voting behavior should take into account a senator’s legislative position, the characteristics of the bills, and the network of influence among the senators.”
“At a technical level,” said Irfan, “the paper uses game theory, machine learning, and AI search techniques.” Furthermore, he believes the political science aspect of the paper made it stand out among the others, which tended to be focused purely on the subject of computer science. “This was only possible because a liberal arts student with multidisciplinary knowledge was involved in this research,” he added.